Transparent Classification for Protest Coding from Images
We present a new method that makes the classification of images more transparent.
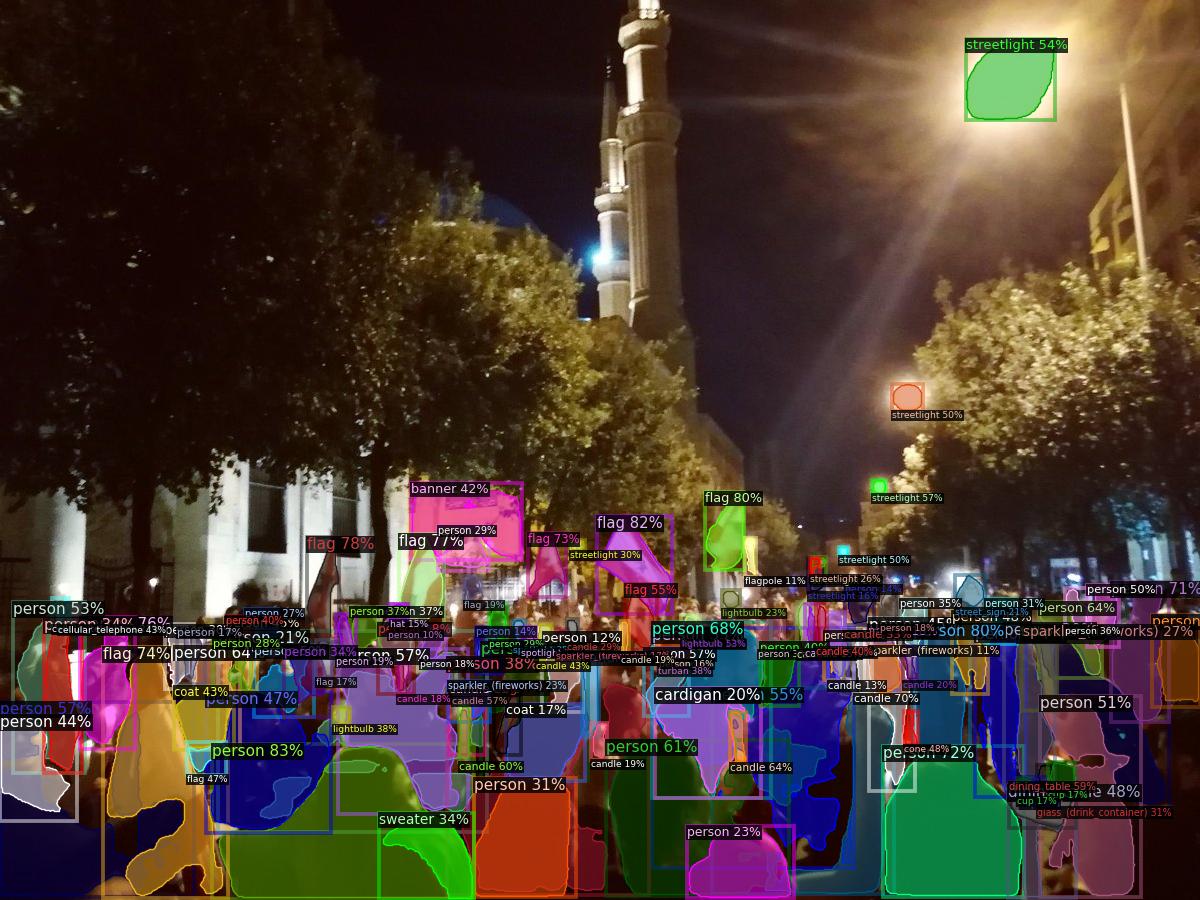
Existing classification algorithms have high accuracy, but they do not provide an explanation of what they use to classify an image. The areas to which the classification algorithm gives a lot of attention still provide the most information, but these areas can hardly be interpreted. Our new method, on the other hand, uses visual attributes that are recognised by a segmentation algorithm, e.g. objects. The set of objects on an image is in turn used to classify an image as a protest image. Thus, the results are based on visual attributes that can be systematically examined and interpreted. This new method allows us to provide insight into what visual attributes are seen in protests. When comparing the visual attributes between different countries, it can also reveal different protest tactics.
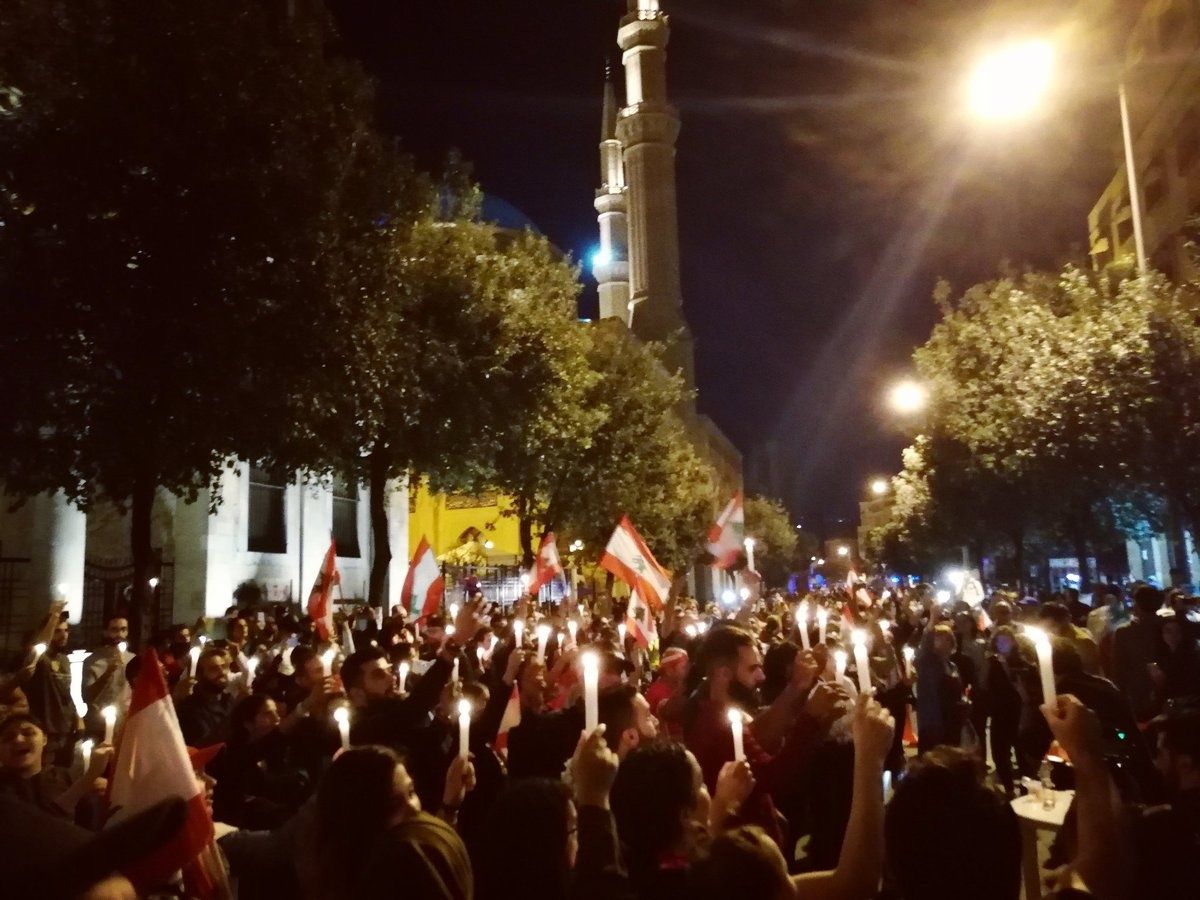
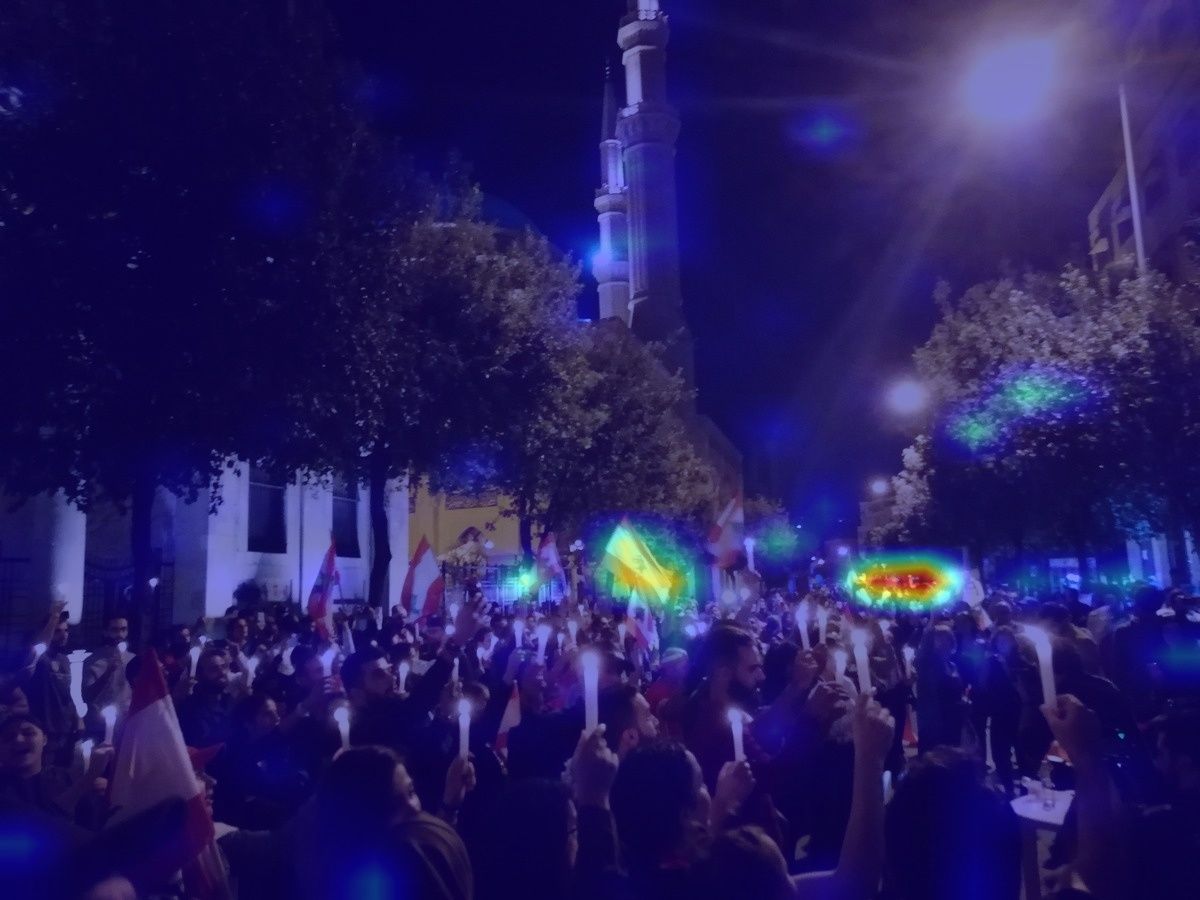
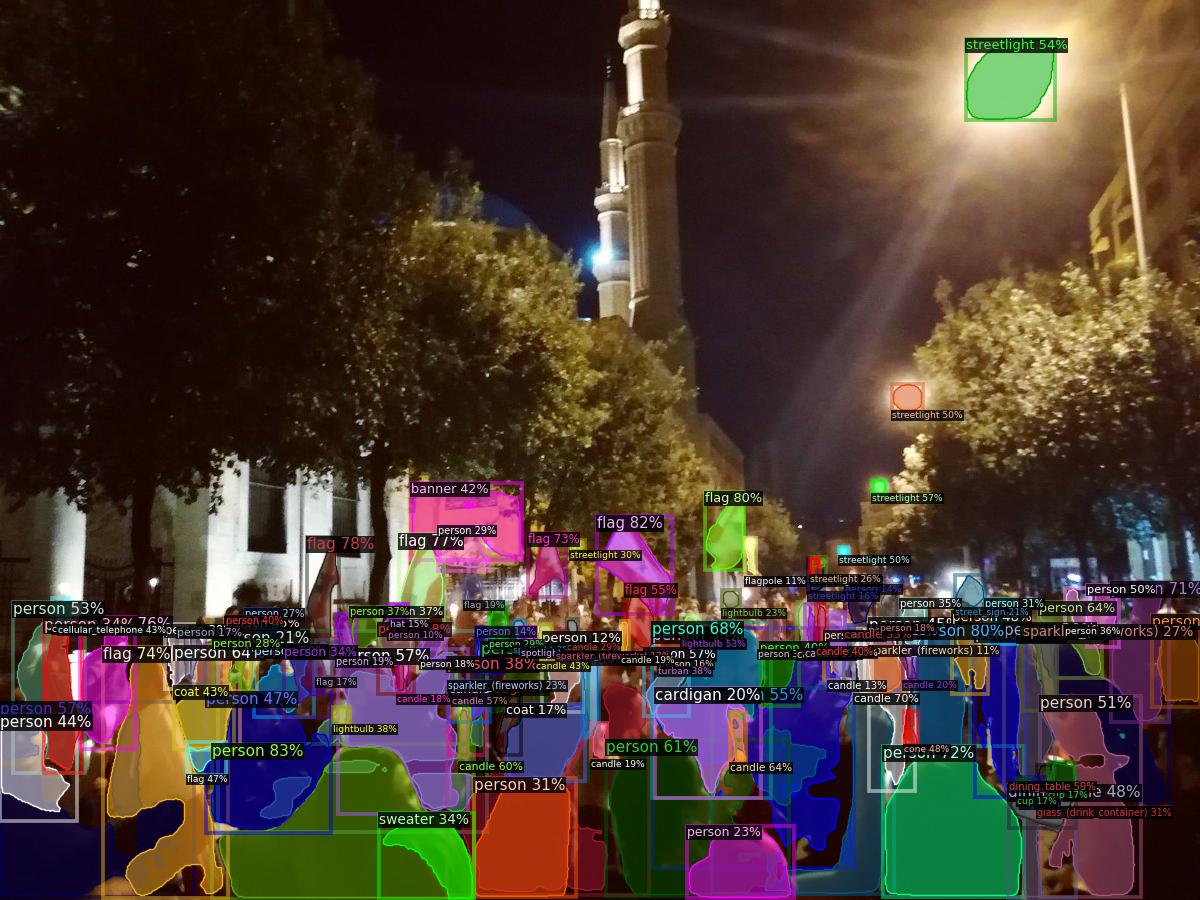
Contributions
We present a transparent method which allows to assess what visual features help the method decide. This approach performs similar to convolutional neural networks. It shows how particular features of protest differ across countries and protest episodes.