Wickedonna
Two activists reported in the Wickedonna blog about protests in China. They collected social media posts for over 74,000 protest events containing images of the protesters, security forces, banners and more. We will improve the prediction of protest in images by using the images from this blog.
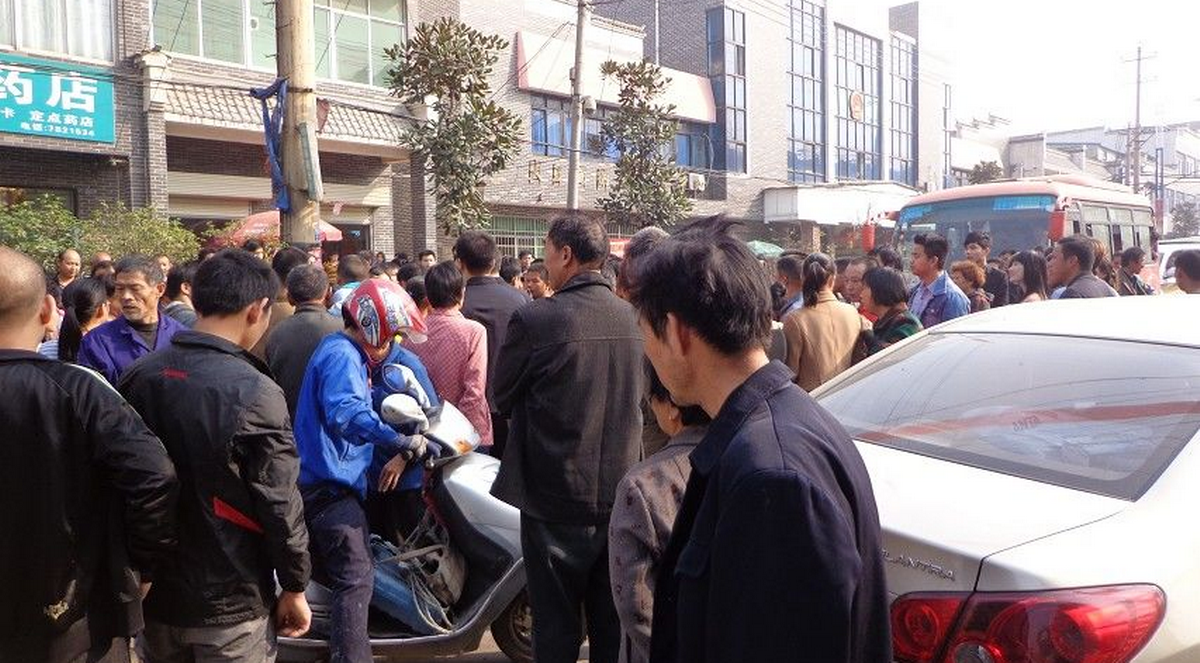
Lack of protest image datasets
Very few images have been collected so far that depict protests. The existing datasets cover mostly images from all types of social events, with protests often underrepresented among concerts, conferences, exhibitions, sports, and theaters. The one or two datasets that specialize in protest events are limited by the small number of protest images though. We are in excited that Christian Goebel and his team at the University of Vienna have annotated a new dataset of protest images. They collected protest images by scraping the images from the Wickedonna dataset. The non-protest images were collected by taking images from Weibo posts (1) whose posts had a low probability of protest based on a text classification and (2) hand-verified. From their data collection, a dataset with about 20,000 protest images and 20,000 non-protest images was obtained. More information about the dataset can be found in his article (referenced below). By using this dataset, our aim is to understand what matters in a protest image dataset.
Predict protest in images
In addition to the problem of images, Christian and his team have already tackled another problem – the prediction of protest. Ultimately, in peace and conflict research, we want to improve our understanding of the emergence, change, and dissolution of political protests. Images are a source of information that has rarely been used yet. Reasons why images have not yet been used are probably because the corresponding methods are still quite new and additionally require large datasets and many computational resources. Christian has nevertheless trained a convolutional neural network, which achieves for the prediction on his validation dataset an accuracy of 92.23%. More information about the model can also be found in his article (referenced below). Our goal is to optimize this existing model and to make the underlying convolutional neural network explainable with its filters and weights.